If there is something I hate to see in presentations is the word “Could” on PowerPoint slides to describe a successful project that uses advanced analytics technologies like Machine Learning. “The pilot was successful. We COULD impact the business by $180M IF we implement the solution”.
This is a sign that a company has tinkered with technology and they are likely miles or light-years away from achieving actual tangible value in a real operationalized production solution.
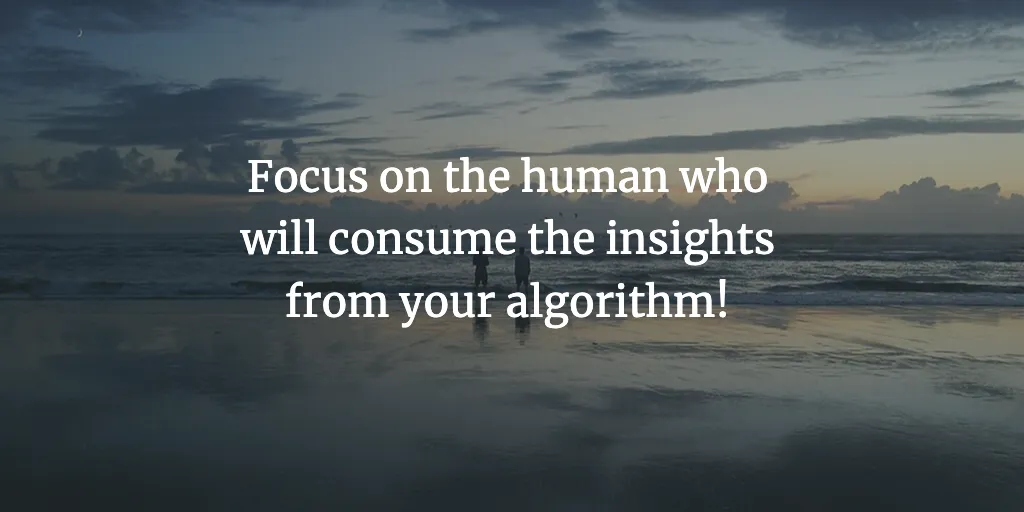
Too many customers, consulting companies, and technology vendors are leading with a technology-first approach to digital transformations that leverage advanced analytics. A customer might request: “I need to incorporate ML into my manufacturing process…” A vendor might push their new and shiny technology or tool which will turn the tide and finally bring success to a project or transformation initiative. You do not need another tool.
The following 4 easy tips will help increase the odds of an initiative that uses Advanced Analytics to deliver real tangible value:
- Start with a clear business case problem that is big enough and strategically aligned for the organization. Ask the customer, if I solve the problem and give you this analytics or prediction, what will you do? How will you act? How will that action bring value to the org? How will you measure the impact of that value? If you do not agree on the way the problem is measured and how the solution will be measured, then you have a huge red flag and you should not start. Ensuring that you are aligned to a big enough problem that is important and strategic is key.
- Work the use case backwards by focusing on the actionability. Who is going to act with the output? How does the analytics or results need to be presented to the end-user in order for them to trust it and act? How many insights or predictions can they handle per day as an example to not be overwhelmed? Focus on the last mile of the problem first and work backwards.
- As you bring the data into the analytics tool, keep the data from the source untouched so that you can control and automate the data cleansing in production. This allows you to configure the needed data transformations into a “production-ready” data pipeline for processing. Constantly asking yourself how this would be transferred to a production system is key to scale quickly past the pilot or POC. At Predikto, we worked our pilots by configuring all the ETL and processing DAGS into a proprietary data ingestion and workflow orchestration system.
- Partner with the lowest level users of the analytics to ensure they are part of the design, test, and learning of the algorithm from the start. Do not walk away to solve the problem and bring it to your customer / end-user with a confusion matrix (precision / recall stats). It is called a confusion matrix because it is very confusing for most people that are not data scientists. You will hit a wall of scepticism and pushback. Bring your end-user or consumer of the analytics along for the ride so they understand the steps. This will improve the chances of the user trusting the analytics and acting on the output
Remember, focus on the actionability in the real world. If users are not acting on the insights, it is another interesting project that would die at PowerPoint with a big use of “COULD” to describe what might have been…